

Based on the previous G*power calculation, I interpret these results as statistically significant and with sufficient statistical power based on the criteria I've specified. Running the PLS algorithm in SmartPLS v.3.2.6 results in four paths with f square values above 0.082 (namely, 0.27, 0.25, 0.23, and 0.10). Type of power analysis: Sensitivity: Compute required effect size - given alpha, power, and sample size Statistical test: Linear multiple regression: Fixed model, single regression coefficient I use the following settings in G*Power 3.1.9.2: I am interested in the statistical power of the predictors' effects on the dependent variable. My dataset has 98 respondents and I have 7 constructs predicting one specific dependent variable. I have a problem relating to the statistical power and critical t boundaries for f square values (effect size). Great to see that there is a thread on G*power here already. With five predictors, this would require you to have a sample size of 248 for a power of 95% to find the 0.2 coefficient significant. If you have an expectation for the standardized coefficient of 0.2 and an overall R² of 0.25, you would get an effect size f² of 0.053. It would require a standardized coefficient of roughly 0.275 if your overall R² is at about 0.5 and a standardized coefficient of 0.336 if your overall R² is at 0.25. Unfortunately, this effect size is not very straightforward to determine. It is the contribution to the R² by the predictor (R²inlcuded - R²excluded) / (1 - R²included). There you have to set the effect size f², which is the same effect size that is reported in SmartPLS under f². Hence, you might want to choose the "Linear multiple regression: Fixed model, single regression coefficient" as the method in G*Power. Many researchers are more interested in the significance of single effects instead of the variance explained by the overall regression equation. Hence, with 138 cases you will find a model with an R² of 0.15 significant at 95% of the time. It assesses the significance of the R² at the given effect size level (e.g., effects size 0.15 = R²). The test above is for the F test of a regression.
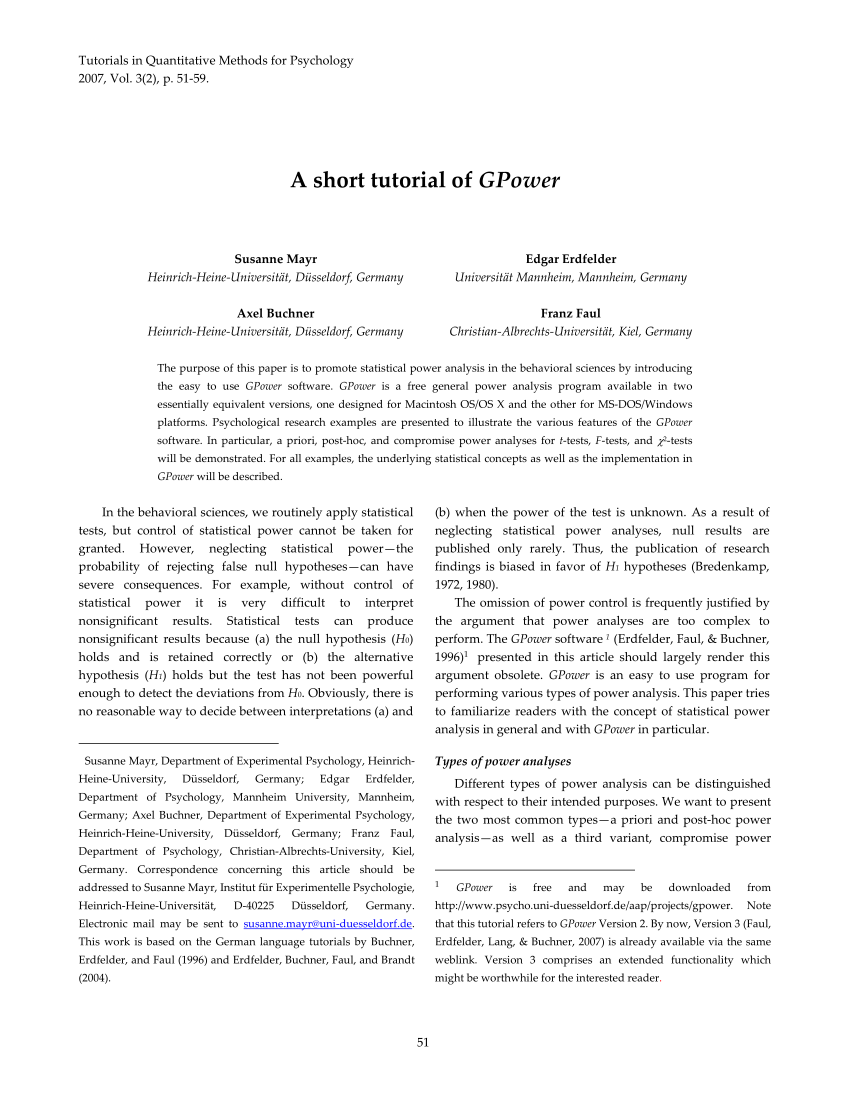
Reporting formats should be standardized so that crucial study parameters could be identified unequivocally.The questions is, which test is the one you are interested in. The field should agree on universally required reporting standards. Publishers and funders should require pre-study power calculations necessitating the specification of effect sizes. Only 14% of highly cited papers reported the number of excluded participants whereas 49% of papers with their own data in 20 reported excluded participants. Only 4 of 131 papers in 2017 and 5 of 142 papers in 2018 had pre-study power calculations, most for single t-tests and correlations. The sample size of highly cited experimental fMRI studies increased at a rate of 0.74 participant/year and this rate of increase was commensurate with the median sample sizes of neuroimaging studies published in top neuroimaging journals in 2017 (23 participants) and 2018 (24 participants). 96% of highly cited experimental fMRI studies had a single group of participants and these studies had median sample size of 12, highly cited clinical fMRI studies (with patient participants) had median sample size of 14.5, and clinical structural MRI studies had median sample size of 50. We evaluated 1038 of the most cited structural and functional (fMRI) magnetic resonance brain imaging papers (1161 studies) published during 1990–2012 and 270 papers (300 studies) published in top neuroimaging journals in 20.
